Introduction
The human brain is the “most complex object in our observable universe,” as Christoff Koch, known for his studies of the neural basis of consciousness, put it. This organ is made up of billions of neurons, synapses, and circuits that govern our thoughts, emotions, and behavior. Neuroscientists have been studying the brain for centuries, trying to unravel its mysteries and understand how it works. These investigations led to the development of artificial intelligence and the idea of creating models that could help us in our tasks. Today, artificial intelligence is on everyone’s lips: news, family conversations, work and, of course, in research. However, with the advent of Deep Learning, a subfield of artificial intelligence, researchers are now able to go further and obtain new answers about the functioning and behavior of our brain. In these pages, we’ll explore how deep learning is revolutionizing neuroscience and transforming our understanding of this highly complex object.
Section 1: What is “Deep Learning”?
Deep Learning (DL) is a specialized area within automatic learning (or, continuing with the terms, machine learning), which at the same time is part of the vast field of artificial intelligence. It is inspired by how our own brain works and aims to replicate that functionality in artificial systems. The central idea of DL is to train what are called artificial neural networks to “learn” from large amounts of data and make predictions or classifications based on them. These networks, designed to mimic the structure and function of the human brain, are made up of interconnected nodes (called neurons) that communicate and process information.
During the training process of a DL model, the available data is divided into two subsets: the training set and the validation set. As its name suggests, the training set trains the model, presenting it with labeled data, which allows the neural network to adjust its parameters and learn the patterns. The validation set, for its part, is used to evaluate the performance of the model during the training process; that is, its generalization capacity.
What sets DL apart from traditional machine learning approaches is its ability to automatically extract relevant features and patterns from raw data. In traditional machine learning, a person is in charge of manually selecting the features. Instead, DL algorithms can autonomously discover and extract useful features as part of the learning process. This ability makes DL especially effective for analyzing complex, unstructured data sets such as images, audio, and text. It has been successfully applied to various tasks, such as image and speech recognition, natural language processing, biological signal analysis, and even autonomous driving.
Section 2: Where can we apply Deep Learning in Neuroscience?
Today, DL models are used (and can be used) in many fields of neuroscience: from neuroimaging or systems neuroscience to gait analysis. The applications, as types of DL models, are endless. In this part, we’ll look at some typical examples that you can apply in your daily research and some promising new approaches that will have an impact on the way we understand the brain.
In the field of neuroimaging, where existing techniques produce a considerable amount of data, artificial intelligence has demonstrated its role in the management and interpretation of brain images and signals. An image is a high-order system that results from the combination of progressively more complex features, ultimately creating a representation of something. Neural networks work in the same way, recognizing these hierarchical elements: first a pixel, then lines, geometric shapes… and saving these elements in their “memory” for learning. One of the most common use cases of DL is diagnostic prediction; discriminate between healthy controls and diseased individuals (Img. 2). Using different magnetic resonance imaging data,(Hu et al. 2022)autism spectrum disorders(Zhang et al. 2022)and even diagnose Alzheimer’s disease and/or mild cognitive impairment(Wang et al. 2017), Parkinson’s disease(Vyas et al. 2022), or cerebrovascular disorders(Liu et al. 2019).
However, the life of a researcher is one in which what is desirable and reality do not usually coincide; common problems include not having a large enough sample size to perform powerful statistics or poor data quality that affects the extraction of relevant features. But, as researchers, we try to improvise, and if we combine this ability with the performance of DL models, solutions emerge to make the most of limited data.
For the case of small data sets, we are going to explain two methods that are often used. The first is the increase in data through generative models (which would be the basis of the “ChatGPTs” that we see now). This technique consists of artificially creating new data by rotating, scrolling, zooming or changing the general style of the images. What is sought with this technique is to increase the sample size by making new copies of the original data set. The addition that DL models provide compared to traditional data augmentation techniques, which iterate over a very reduced spectrum, is to create synthetic images that are different from the original ones, maintaining the essential characteristics of the real images.(Perez and Wang 2017). The second method is called “dropout”. The idea behind it is to introduce random noise into specific layers of a neural network, in such a way that the neurons in those layers are “masked” or eliminated. Using this method, the model can obtain a result faster, by obtaining the most robust or generalizable features.(Audhkhasi, Osoba, and Kosko 2016;Srivastava et al. 2014)that can be shared by the remaining neurons, an especially important feature if we are talking about small data sets.
The quality of neuroimaging data is one of the most important aspects for a critical interpretation of images. Some techniques, such as diffusion-weighted magnetic resonance (DW-MR), rely on complicated estimation methods that have remained unchanged for many years. The new approaches suggest that more advanced DL models could perform better in estimating clinical images, reducing the errors of previous standard methods.(Karimi and Gholipour 2022).
Finally, let’s look at some examples in the field of systems neuroscience. This term encompasses a series of study areas related to the function of neural circuits and systems, such as memory or language. These (non-artificial) neural networks are complex systems in which there are interactions between layers and with other structures, and some mechanisms remain unknown. During these last few years of impressive development of artificial neural networks, scientists have attempted to replicate structures in the brain, building models that explain neural recordings and provide highly accurate spatial representations.
If we think about language in the brain, there has long been a hypothesis called predictive coding, according to which the brain’s language system is optimized for future processing. In this way, the brain would be constantly generating and updating a “mental model” of the environment and these predictions created by the model would be compared with the actual input signals from the senses, adjusting them. In keeping with this hypothesis, the researchers developed a model that could predict human language processing neural responses almost 100%!, mimicking how predictive language processing is implemented in neural tissue.(Schrimpf et al. 2021).
Closely related to language, memory has also been a field of study for artificial intelligence. Some researchers, seeing that many DL neural networks are based on brain networks, decided to take a closer look at memory formation. It is well known that the hippocampus plays a vital role in our memory processes. It is often described as a key player in forming new memories and consolidating them for long-term storage, but not indefinitely. When we experience something new, the hippocampus helps encode and process these memories.
Wittington and his colleagues conducted a curious experiment on memory. First, they trained a DL model to learn spatial representations, showing that the model replicated what hippocampal cells did. This allowed them to move on and they decided to rewrite the mathematical foundations of a well-known neuroscience model: “The Tolman-Eichenbaum Machine” (TEM), which replicated the hippocampus phenomenon. The TEM is a hypothetical model that combines the work of Edward Tolman and Gary Eichenbaum. The first proposed cognitive map theory, suggesting that animals, including humans, form mental representations of their environment. Eichenbaum, for his part, extensively studied the hippocampus and its involvement in memory processes. According to TEM, the hippocampus functions as a ” in which individual cells or groups of cells represent different aspects of the environment and associated memories. Something like the hippocampus creating a map of the environment in its cells. Wittington and his team then combined the worlds of AI and neuroscience and created the TEM-t (which from the name sounds like an advanced version of a Terminator), a much more efficient and capable model. in which individual cells or groups of cells represent different aspects of the environment and associated memories. Something like the hippocampus creating a map of the environment in its cells. Wittington and his team then combined the worlds of AI and neuroscience and created the TEM-t (which from the name sounds like an advanced version of a Terminator), a much more efficient and capable model.(Whittington, Warren, and Behrens 2021).
So what are the neuroscientific implications? It is a fairly accepted hypothesis that the TEM reproduces the phenomenon of the hippocampus. If t-TEM can do even better, this means that DL’s model could hide some explanations for how neurons work at the level of the hippocampus.
Thus, observing the artificial neurons could help us understand the real ones.End of the form
Can you help us to become more? Become a member and participate. Spread our word on the networks. Contact us and tell us about yourself and your project.
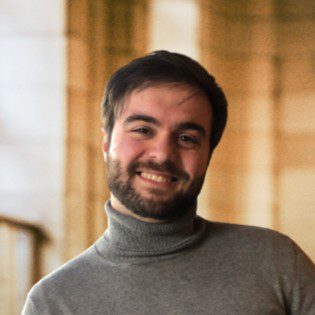
Alfonso de Gorostegui
PhD Candidate in Neuroscience
Hola! Mi nombre es Alfonso de Gorostegui y estoy en camino de terminar mi doctorado en Neurociencia con la Universidad Autónoma de Madrid, en concreto en el estudio de marcha de pacientes con enfermedades neurológicas.